Esports research ideas: Patch analyser
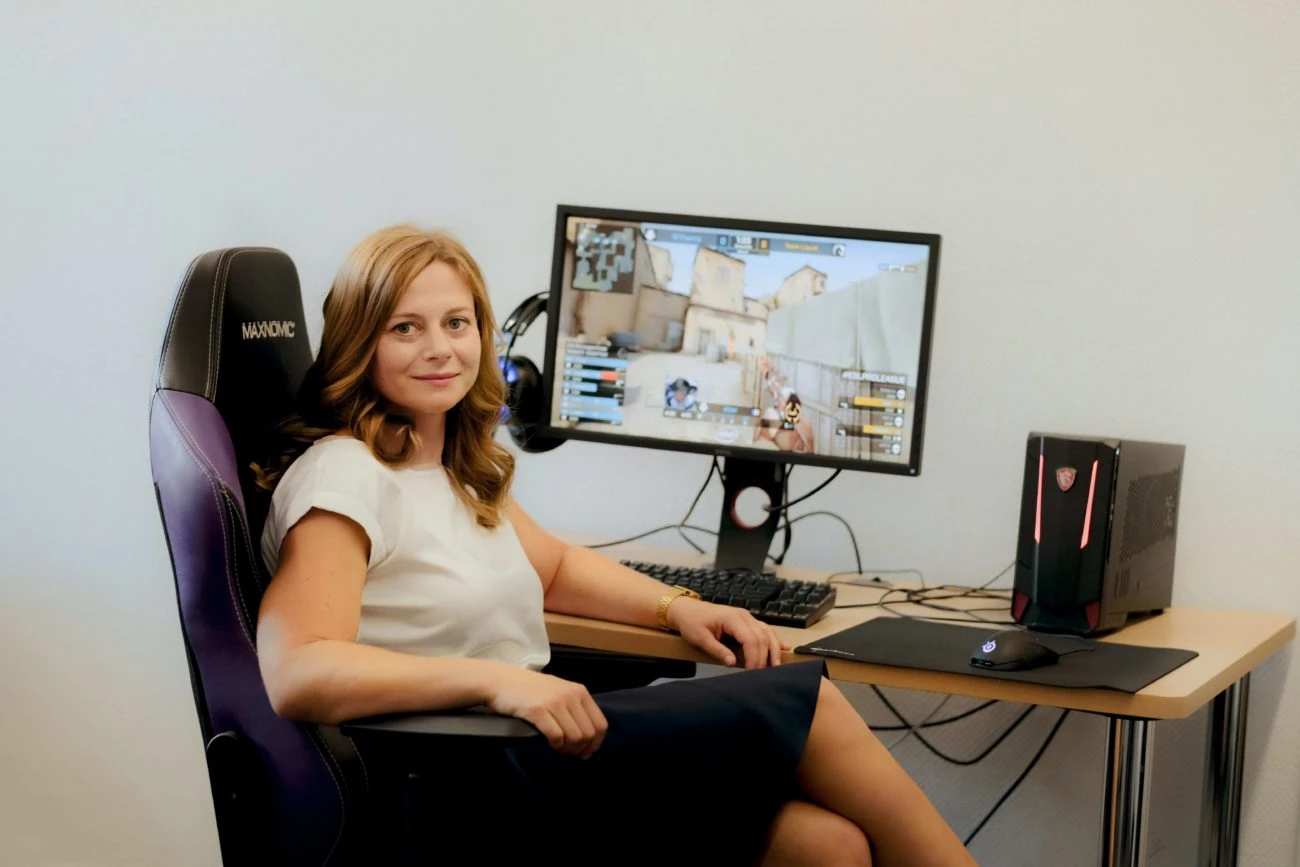
We all know patches from our phones and computers – software needs to be updated from time to time, either to enable new functionality or to patch existing problems.
In esports, the significance of patching goes way beyond that – particularly in MOBAs, patches are often introduced specifically to shake things up.
A single patch can eliminate a building type, introduce new heroes, or change the old heroes so much that they are barely recognisable.
There are also subtle, but important changes going on: A cool-down on an ability might drop by 5% or an item’s price might be tweaked.
How do these patches affect the gameplay? It’s hard to nigh impossible to say right away. Usually companies like Bayes Esports need to wait and see how professional teams adjust to the changing game rules and then follow their lead. A new “meta” game eventually develops – until the next patch goes live.
While this is what keeps the game interesting and fresh for spectators and players, it presents a significant challenge to anyone who wants to create betting odds.
Side markets for MOBAs focus on objectives such as killing certain monsters, and these monsters’ properties and priority changes quite often with patches. If you just let your models run untouched, you’re exposing yourself to any number of clever punters.
The problem with fixing models, however, is that it takes time to generate new data. We cannot adjust to what we do not know – and we do not know how a patch will affect the meta. Sure, there are expert analysts dissecting every patch and offering their predictions, and human traders can often take over for a time, but human experts err often.
Depending on how busy the season is, it can take up to several weeks to gather enough data to assert model quality for side markets. This is valuable time during which side markets cannot be offered or can only be offered at a higher risk.
Now imagine a piece of software that is fed patch notes and is able to predict meta game changes. This would be a game changer in the industry! There are several ways that this could be made possible, with self-play being at the top of my list.
What if a computer steered two League of Legends or DotA2 teams who played against each other until they developed a new meta?
Then we could compare the shifts in strategies and collect statistics from the games just like we would from real matches and use these to make new prediction models. Except that where we currently need to wait a week or two to collect enough relevant matches from top teams, we would now need mere hours.
This is not as futuristic as it sounds. OpenAI has already succeeded at teaching a machine to play Dota2 on a professional level. Modern AI is able to learn strategies, coordinate a team and optimise for long-term goals.
Going from here to exploring patches is still a major step, but it’s a step that, in my opinion is reasonable and can be taken. It just needs a team dedicated to this task who has access to the best quality data like Bayes Esports provides. Who knows, maybe already a year from now we’ll be looking to an AI assessment of patch notes instead of the traditional expert opinion?
Dr Darina Goldin is the director of data science at Bayes Esports. She started playing competitive Team Fortress 2 in grad school. While no longer an active gamer, she is still an avid Esports fan. At Bayes, she has created numerous predictive models for Counter Strike, Dota2, and League of Legends. When not crunching numbers, you can find her at the gym training Brazillian Jiu Jitsu.